AI - Game Changer for Data Management
Data classification can feel like trying to sort through a mountain of paperwork without a filing system. So why is it such a complex and time-consuming task? Picture this: you’re managing a bustling email server flooded with millions of messages daily—work documents, travel itineraries, event invitations, and even birthday greetings. Sifting through these to find what matters can be like searching for a needle in a haystack. That’s where AI steps in and changes the game.
1. Why Traditional Data Classification Falls Short
Conventional data classification methods are typically manual and rules-based. While these approaches might work for small datasets, they crumble under the weight of modern data volumes. Manual sorting is not only slow but also prone to human error. Rules-based systems, on the other hand, can’t keep up with the nuances and ever-changing nature of data. These limitations lead to inconsistencies, compliance risks, and inefficient data management practices that can stifle business growth.
DID YOU KNOW
In 2024, around 15% of all UK businesses, equating to 432,000 companies, have already adopted at least one AI technology for operations such as data management and analysis.
Gov.uk
2. Enter AI - The Data Classification Superhero
AI transforms data classification into a seamless, automated process. Imagine having a team of tireless assistants who can instantly scan through every piece of data—whether it’s an email, document, or spreadsheet—and immediately understand its content. Is it an invoice? A confidential report? A simple reminder? With AI, this task, which could take a human team days to complete, is done in seconds.
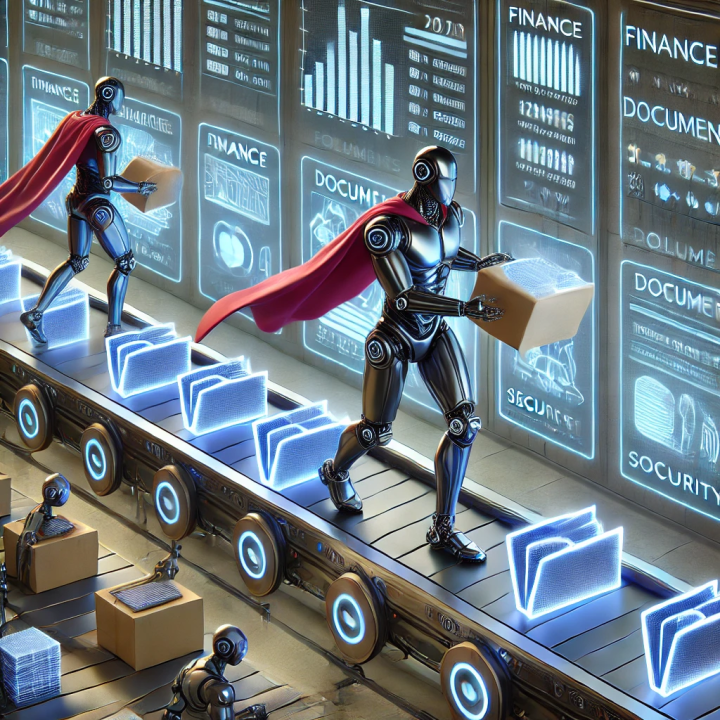
AI achieves this by using advanced algorithms and natural language processing (NLP) to recognise patterns, understand context, and categorise data with incredible accuracy. It doesn’t just identify keywords; it comprehends the meaning behind the text, differentiating between similarly worded information based on context.
3. How AI-Based Data Classification Works
AI data classification involves a series of steps that allow it to automatically analyse and categorise massive datasets based on specific criteria. Here’s a quick breakdown of how it works:
a. Data Analysis and Categorisation
AI scans the content, context, and metadata of each file to identify what type of data it is—personal information, financial records, contracts, etc.
b. Pattern Recognition
Using machine learning models AI identifies patterns within the data and learns to differentiate between various categories even when the content is ambiguous or new.
c. Real-Time Classification
AI can handle real-time data streams, ensuring that as new data flows in, it’s classified instantly according to pre-defined rules or adaptive learning mechanisms.
Continuous Learning and Improvement: AI learns and adapts over time, improving its classification accuracy based on new data and user feedback.
This intelligent, automated approach not only saves time but also ensures consistency and compliance, making data classification more reliable than ever before.
AI data classification involves a series of steps that allow it to automatically analyse and categorise massive datasets based on specific criteria. Here’s a quick breakdown of how it works:
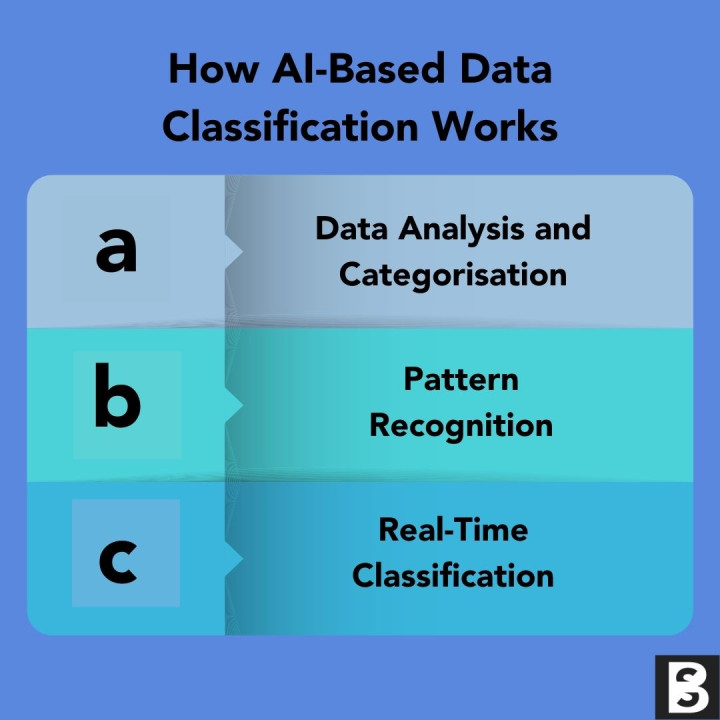
4. Training the AI - The Foundation of Successful Data Classification
Even the most sophisticated AI models require well-structured, high-quality data to perform accurately. This process involves preparing the data to ensure it’s clean, properly labelled, and representative of all the categories you want the model to recognise. If not handled correctly, you risk a phenomenon known as “garbage in, garbage out,” where poor-quality data leads to inaccurate classifications.
5. A simplified version of the training process:
a. Data Collection and Assessment
Identify relevant data sources and assess them for inconsistencies, biases, and completeness.
b. Data Labelling and Validation
Define categories and label data points accurately, either manually by experts or through automated labelling tools.
c. Address Data Quality Issues
Handle missing values, outliers, and any data imbalances to ensure the model has a solid foundation to learn from.
d. Feature Engineering
Create new features or attributes that make classification easier for the AI, such as converting text data into numerical representations.
e. Choosing the Right Algorithm for the Job
Selecting the right AI algorithm is key to building an effective data classification model. Different algorithms excel in different scenarios. Here’s a snapshot of a few popular choices:
f. Logistic Regression
Great for simple binary classification but struggles with complex, non-linear data.
g. Decision Trees
Easy to interpret but can be prone to overfitting if not managed correctly.
Support Vector Machines (SVM): Powerful for high-dimensional data, yet computationally intensive.
h. Naïve Bayes
Efficient for large datasets but assumes features are independent, which might not always be true.
i. K-Nearest Neighbour's (KNN)
Simple and effective for some tasks, but its performance can drop with high-dimensional data.
Choosing the best-fit algorithm depends on factors like the number of categories, data imbalance, complexity, and the need for interpretability.
6. Types of AI Learning Approaches for Data Classification
Broadly speaking, AI data classification models fall into three learning categories:
a. Supervised Learning
The AI is trained using labelled data (data with known outputs), making it ideal for image classification, spam filtering, and predicting outcomes based on historical data.
b. Unsupervised Learning
The AI identifies patterns in unlabelled data, making it suitable for clustering and anomaly detection.
c. Reinforcement Learning
The AI learns through trial and error, receiving feedback in the form of rewards or penalties to optimise decision-making over time.
Each approach has its strengths and is suited to different types of data classification problems.
7. Evaluating and Optimising Your AI Model
Once your model is up and running, the next step is evaluating its performance. Metrics like accuracy, precision, recall, and F1-score provide insights into how well the model is performing. It’s crucial to test the model on unseen data to gauge its ability to generalise to new scenarios. If the model struggles in certain categories, tweaking the data or trying different algorithms can lead to better results.
It’s also important to ensure that the model is not biased or overfitting to specific categories. Regular monitoring, fine-tuning, and updating the model based on new data inputs will keep it effective and relevant.
8. The Business Impact - Why AI-Based Data Classification is a Game-Changer
AI-powered data classification offers more than just automation—it brings strategic advantages:
a. Boosts Efficiency
Eliminates manual data handling, freeing up employees to focus on higher-level tasks.
b. Enhances Security
Automatically identifies and protects sensitive information, reducing the risk of data breaches.
c. Ensures Compliance
Maintains adherence to regulatory requirements with detailed audit trails and real-time classification.
d. Improves Decision-Making
Provides timely access to accurate data, enabling data-driven decisions.
e. Partnering for Success
Building and maintaining a successful AI-based data classification system requires expertise in both data science and business strategy. For many organisations, partnering with experienced AI specialists is the best approach.
DID YOU KNOW
In 2020, UK firms spent £16.7 billion on AI. This figure is expected to more than double to £35.6 billion by 2025 and could reach as high as £127 billion by 2040, depending on adoption rates and advancements in AI capabilities
Gov.uk
Our team offers end-to-end solutions, from data preparation, algorithm selection and optimisation.
Let’s talk about how AI can simplify your data classification processes and transform your business. Reach out to our team today!
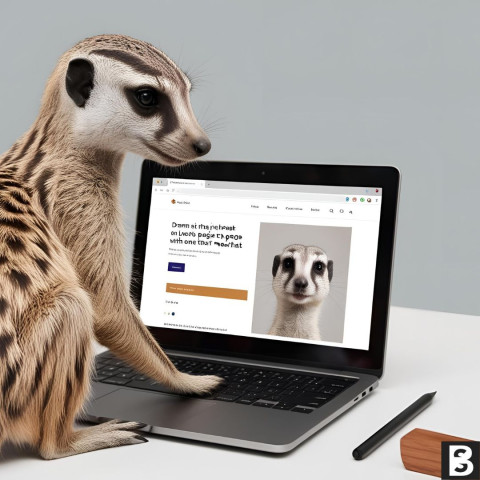
Website Design Trends for 2025
Introduction The world of website design is in a constant state of flux,...
8 min read
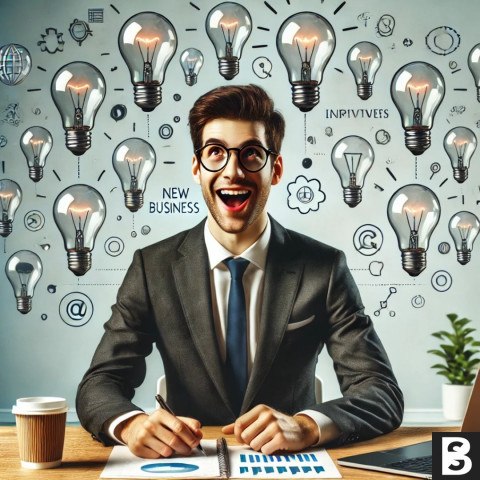
New Business Start-up Checklist 30 Key Considerations
Contents Assess Your Strengths and Weaknesses Define the Need Evaluat...
24 min read
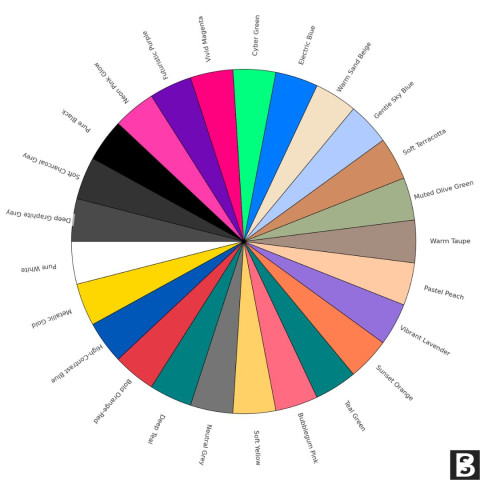
Web Design Colour Trends for 2025
Contents Digital Tranquility (Earthy & Muted Tones) Futuristic Neons (C...
4 min read